The Impact of Machine Learning Data Annotation on Business Efficiency
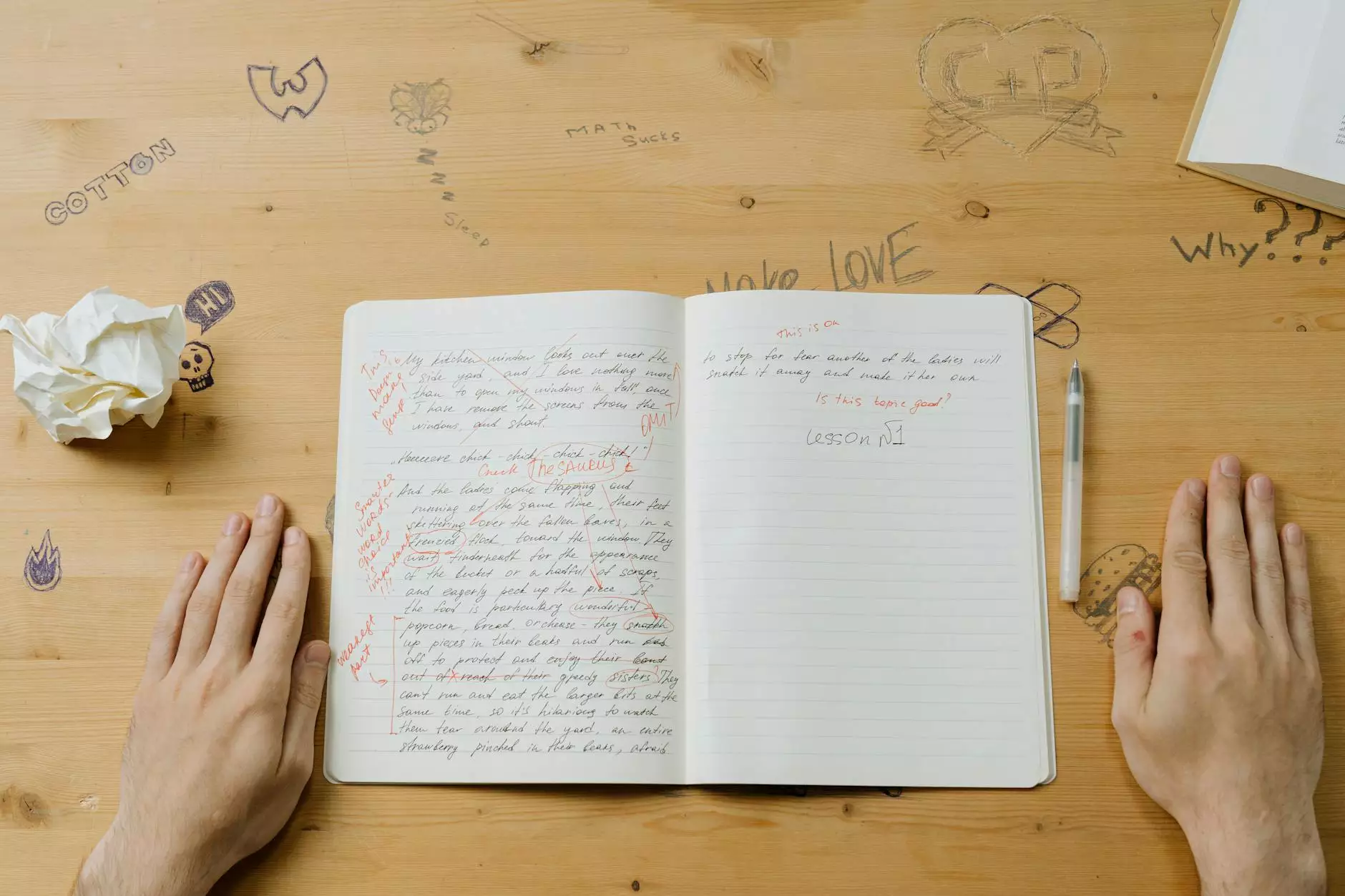
Machine learning data annotation has emerged as a critical process for organizations seeking to leverage artificial intelligence (AI) effectively. In the context of businesses within the home services, keys, and locksmiths sectors, the integration of this technology can significantly impact operational efficiency, customer satisfaction, and overall success.
Understanding Machine Learning Data Annotation
Machine learning data annotation is the process of labeling data to enable machine learning algorithms to learn from it. By providing accurate annotations, businesses can train their AI systems to recognize patterns, make predictions, and enhance decision-making capabilities. This is particularly essential for industries that rely on precise data analysis and responsiveness to customer needs.
What is Data Annotation?
Data annotation involves marking or tagging data, such as images, text, or audio, to make it understandable for machine learning algorithms. This process is crucial because unsupervised machine learning algorithms require a well-defined dataset to learn effectively. There are different types of data annotation, including:
- Image Annotation: Tagging images for object recognition.
- Text Annotation: Annotating text for sentiment analysis, entity recognition, etc.
- Audio Annotation: Labeling sounds for speech recognition systems.
The Role of Annotation in Business
In the business world, especially in sectors like home services and locksmith operations, machine learning data annotation plays a vital role. Companies are increasingly utilizing AI to improve customer engagement, streamline operations, and enhance service delivery. Here’s how data annotation contributes to these processes:
1. Enhanced Decision Making
With comprehensive data annotation, businesses can analyze various aspects of their operations. For locksmiths, machine learning can predict customer needs based on seasonal trends and historical data. Accurate data interpretation leads to better timing for promotions and service offerings.
2. Improved Customer Service
Machine learning models that utilize annotated data can assist in developing chatbots and virtual assistants. These tools can understand and respond to customer inquiries more effectively. For example, by analyzing past customer interactions, businesses can create a more personalized experience for clients seeking locksmith services.
3. Optimized Marketing Strategies
By leveraging machine learning data annotation, businesses can better understand customer demographics and preferences, leading to optimized marketing strategies. Tailored advertising based on annotated data insights ensures that locksmith services reach the right audience promptly, increasing conversion rates.
The Process of Machine Learning Data Annotation
Implementing machine learning data annotation within a business requires a systematic approach. Here’s a look at the typical process:
- Data Collection: Gather relevant data types that pertain to business operations.
- Annotation Guidelines: Develop guidelines to maintain consistency and accuracy during the labeling process.
- Annotation Tools: Utilize specialized software tools for efficient data labeling.
- Quality Assurance: Implement review mechanisms to ensure high-quality annotations are maintained.
- Model Training: Use the annotated data to train machine learning models.
Technological Advancements in Data Annotation
The landscape of machine learning data annotation is continually evolving. Emerging technologies such as artificial intelligence and automation tools are streamlining the process. Here are some advancements making an impact:
1. Automated Annotation Tools
Automated tools can significantly reduce the time and cost associated with data annotation. By leveraging AI algorithms, these tools can quickly and accurately label datasets, allowing businesses to focus on strategic tasks rather than manual classification.
2. Crowdsourcing Annotation
Many companies now utilize crowdsourcing to annotate large datasets. This method allows businesses to access a diverse pool of talent and accelerate the annotation process, which is invaluable for home service providers looking to implement machine learning solutions promptly.
Challenges in Data Annotation
While there are various benefits associated with machine learning data annotation, companies may encounter challenges, such as:
- Volume of Data: The sheer amount of data can be overwhelming, requiring robust systems to manage it effectively.
- Quality Control: Ensuring the quality of annotated data is crucial; even minor errors can lead to significant issues in model training.
- Domain Expertise: Specific industries such as locksmith services may require domain experts to conduct proper annotations, which can be hard to find.
Best Practices for Effective Data Annotation
To mitigate challenges and optimize outcomes, businesses can adopt several best practices when engaging in machine learning data annotation:
1. Establish Clear Annotation Guidelines
Having well-defined guidelines will ensure consistency across the annotation process. Clear instructions help annotators understand expectations, reducing variability in labeling.
2. Invest in Training for Annotators
Training programs for annotators are crucial, especially in specialized fields like locksmith services. Ensuring that annotators are knowledgeable in the subject matter will lead to higher quality annotations.
3. Utilize Technology Wisely
Implementing advanced annotation tools, including AI-based software, can enhance efficiency. Additionally, investing in quality assurance technologies ensures that the data maintains high standards, supporting better machine learning outcomes.
The Future of Machine Learning Data Annotation
The future of machine learning data annotation within the business landscape looks promising. As technology develops, we can anticipate:
1. Greater Integration of AI
As AI continues to advance, its integration into data annotation processes will streamline operations further, leading to quicker turnarounds and more sophisticated models.
2. Enhanced User Experiences
In fields like home services and locksmith operations, personalized user experiences will become increasingly common. Leveraging data insights, services will adapt to individual customer needs, fostering loyalty and satisfaction.
Conclusion
The importance of machine learning data annotation cannot be overstated. For businesses in the home services sphere, particularly locksmiths, the capacity to harness this technology is revolutionary. It not only enhances operational efficiency but also leads to improved customer satisfaction and data-driven decision-making.
As companies continue to embrace AI and machine learning, those that prioritize quality data annotation will undoubtedly rise to the forefront of their industries, setting themselves apart as leaders and innovators.